This post, co-authored by Paul Belleflamme and Martin Peitz (whom IPdigIT is very happy to welcome in its team!) is an excerpt of a book, entitled ‘The Economics of Platforms‘, that the two authors are currently preparing for Cambridge University Press.
As already explained on this blog (se, e.g., here and here), network effects arise when the users of a solution (product, service, platform, …) care about participation and usage decisions of other users when taking their own decision. Network effects are positive if the value of the solution for each user increases the more users there are.
In the current economic and management literature, it is customary to distinguish between two categories of network effects, according to whether the users whose decisions influence one another belong to a unique group or to separate groups. If all users belong to a unique group, network effects are said to be ‘within-group‘: more usage within the group directly affects each group member; communication devices are the prototypical example. But there are also many environments in which users in one group mainly care about the participation of users belonging to another group. This is so on many digital platforms: BlaBlaCar’s value for drivers increases when more passengers use the platform, and vice versa; similarly, authors on this blog enjoy a larger audience and we hope that you, readers, enjoy a larger set of authors. In these situations, network effects are said to be ‘cross-group‘.
The previous examples suggest that there is a clear dividing line between these two types of network effects. However, there exist many environments in which it may be tricky to determine whether the users of a platform belong to the same group or whether they should be split into separate groups. The objective of this post is to illustrate why it may be difficult to categorise network effects, and to discuss why it does—or does not—matter.
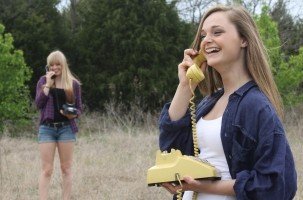
Who’s calling?
Telecommunication networks provide a nice illustration of the difficulty to draw a clear line between within- and cross-group network effects. Most of the economic literature on telecommunication networks assumes, for simplicity, uniform calling patterns, i.e., an equal likelihood for each subscriber to call and be called by any other subscriber.1 This assumption of fully symmetric participants implies a single group exhibiting within-group network effects. Another simplifying assumption would be to consider that some people only make calls, while others only receive calls (e.g., restaurants and customers who want to order for delivery or make a reservation); in that case, there would be two distinct groups, with only cross-group network effects. The reality is naturally somewhere between these two extremes: subscribers are heterogeneous in their propensity both to make calls and to receive calls. Moreover, calling patterns are largely reported to be nonuniform: most subscribers have a ‘calling circle’, i.e., a subset of subscribers with whom they interact more frequently than with others.2 Seen from an individual subscriber’s perspective, network effects are then mostly within-group (i.e., inside the calling circle), cross-group network effects (i.e., outside the calling circle) being relatively limited; yet, each subscriber makes a different distinction between the two types of network effects as calling circles differ.
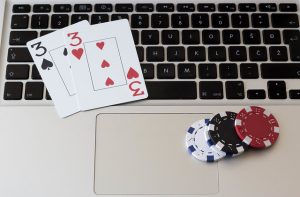
Poker face … or poker faces?
Online games provide another environment for a platform with, at first sight, a single user group—in this case, the players—that is subject to network effects; yet, after a closer look, one observes a richer structure, featuring within-group and cross-group network effects, as illustrated by survey evidence of cross-group network effects in online poker.
Wimmer, Philander and Redona (2018) ran a survey among players of online poker in which they asked them to evaluate the presence of other players on the attractiveness of a poker site.3 Clearly, the more players are active on a site, the shorter the waiting time to a play. This suggests that there are positive within-group network effects. However, a closer look reveals that players are heterogeneous in their abilities. Poker, as all zero-sum games in which success depends on players’ abilities, has the property that winning probabilities increase in the ability of a player and decrease in the ability of other players. If we distinguish between low-skilled and high-skilled players, then every player finds a site more attractive if there are more low-skilled players. If the reduced waiting time from a larger pool is dominated by the reduced probability of winning, then every player finds a site less attractive if there are more high-skilled players. Thus, evaluated in a mixed pool, we would expect that there are positive within-group and cross-group network effects generated by low-skilled players and negative within-group and cross-group external effects generated by high-skilled players. In line with this hypothesis, the authors find that more participation by low-skilled players makes the poker site more attractive, while participation by high-skilled players does the opposite.
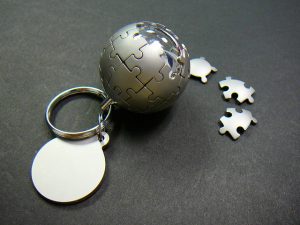
“Readers make writers and writers make readers”4
Since people can read and write on Wikipedia and no other users re involved, one may suspect that there is a single user group. However, some users are almost exclusively readers, whereas others may frequently update and add content. Thus, also in this case we can distinguish different groups, contributors and non-contributors. Natural experiments are an interesting way to gain insights into the presence and type of within-group and cross-group network effects. A natural experiment takes place if a system consisting of two or more groups that are linked through network effects is exposed to a shock that directly affects the user base of one or more groups.
Wikipedia is an example of successful private provision of a public good. While one may expect that Wikipedia suffers from the free-rider problem—that is, people are less willing to contribute the larger the number of users, Zhang and Zhu (2011) find the opposite using data on Chinese Wikipedia in 2005.5 The shutdown of Wikipedia in mainland China in October 2005 allows them to analyse the response by contributors from other places (e.g., Taiwan, Hong Kong, Singapore) to this shock in readership and number of contributors. Since the number of potential contributors has dropped, the free-rider problem suggests that non-blocked users are more likely to contribute. By contrast, Zhang and Zhu find that contribution levels of non-blocked users dropped by more than 40 percent with the shutdown. As they argue,
“contributors receive social benefits from their contributions, and the shrinking group size reduces these social benefits.” (p. 1601)
Distinguishing between contributors and non-contributors, this suggests that there are positive cross-group network effects from non-contributors to contributors. Clearly, we also expect positive cross-group network effects from contributors to non-contributors to be present, as the latter benefit from improved content on Wikipedia.
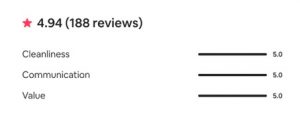
Union is strength
The previous examples show that many platforms that may be thought to cater to a single group of users are better described, upon closer examination, as serving multiple groups because of heterogeneous network effects. On the other hand, one should also note that if a platform facilitates the interaction between two (clearly distinct) groups of users, within-group and cross-group network effects are present and may be difficult to disentangle. Take the example of peer-to-peer marketplaces like Uber or Airbnb, which enable the interaction between providers and consumers of services; clearly, each group exerts positive cross-group network effects on the other group. Yet, the quality of the matching between peers from the two groups increases with the volume and reliability of data that the platforms collect from providers and consumers alike. Hence, also a form of within-group network effects appears: the larger the participation on one side, the more data is generated (about feedbacks, reputation, reviews, geo-localisation, etc.), which enhances the
quality of the platform’s service and, thereby, the utility of all users on the same side.
Does it matter?
In a nutshell, the distinction between within- and cross-group network effects may be hard to establish in some environments. This may not be a problem for a number of issues. For instance, if we are concerned with the evolution of competition among platforms, we understand that positive network effects–whether within or mutual across groups–generate a self-reinforcing process (big platforms tend to become bigger, while small platforms tend to become smaller). As previously explained on this blog, such self-reinforcing process may lead to winner-takes-all situations.
Yet, determining the exact nature of the network effects may prove much more crucial when it comes to design specific strategies. In the poker example, for instance, a platform that considers that network effects are of the within-group kind would deploy strategies to attract any player, irrespective of their skills. Yet, such strategy may lead to an excess of high-skilled players, which may eventually reduce the attractiveness of the platform for any player. From that point of view, it is key to identify more precisely the types of network effects that link users on the platform.
1 See, e.g., Armstrong, M. (1998). Network Interconnection in Telecommunications. Economic Journal 108, 545–564 / Laffont, J.-J., P. Rey, and J. Tirole (1998a). Network Competition: I. Overview and Nondiscriminatory Pricing. Rand Journal of Economics 29, 1–37 / Laffont, J.-J., P. Rey, and J. Tirole (1998b). Network Competition: II. Price Discrimination. Rand Journal of Economics 29, 38–56 / de Bijl, P. and M. Peitz (2002). Regulation and Entry into Telecommunications Markets. Cambridge: Cambridge University Press. 2 See, e.g., Hoernig, S., R. Inderst and T. Valletti (2014). Calling Circles: Network Competition with Nonuniform Calling Patterns, Rand Journal of Economics 45, 155–175. 3 Wimmer, B. S., Philander, K. S., and Redona, M. (2018). The Effects Network Externalities on Platform Value and Management: Evidence from Internet Poker Users. Available at SSRN. 4 Quote attributed to Carl McKever in Poetic Puberty: Developmental Stages of a Poet. 5 Zhang, X., and Zhu, F. (2011). Group Size and Incentives to Contribute: A Natural Experiment at Chinese Wikipedia, American Economic Review 101, 1601–1615.
Photo credits: 1. Visualhunt / 2. marcoverch on Visualhunt.com CC BY / 3. @bastique on Visualhunt.com CC BY-SA /